About the challenges of sovereign risk modelling
This article was originally published on 6 December 2016 on LinkedIn.
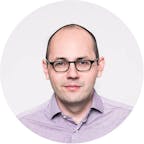
Bernhard Obenhuber
Aug 24, 2018
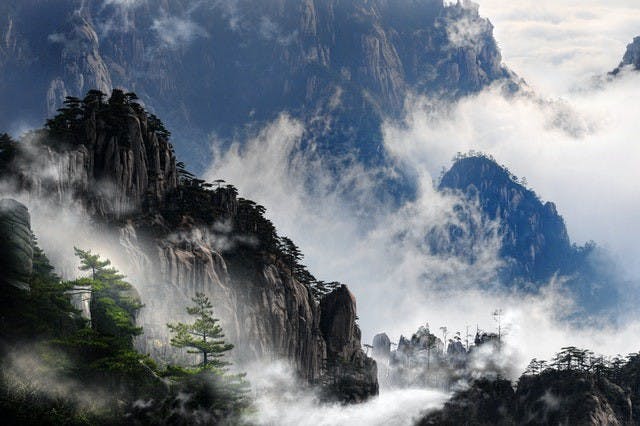
At CountryRisk.io we talk to many private and public sector companies about country and sovereign risk analysis; how to define country and sovereign risk; how to measure it; how to model it. In this post we want to share some thoughts and experiences on the modelling part.
My co-founder Jennifer Asuncion and I have spent many years using various ways to assess country/sovereign risk. Our experience has been mainly in the context of credit risk exposure management within large financial institutions and as an input factor for investment management.
We worked in large banks, which utilized the internal rating based approach for Basel regulation. On the investment management side, the need for diligent assessment of sovereign risk has become ever more critical in recent years. In the past, the assessment of sovereign risk — from the likes of Argentina’s endless restructuring saga to Cote d’Ivoire’s default, or in assessing the impact of conflict between Ukraine and Russia — was left by and large to the emerging markets research teams. Within the context of global asset allocation decisions, such emerging market country risks seem trivial, given that emerging markets are still just roughly 5% of global investment portfolios. A restructuring in Mozambique or Belize has widespread dire economic and financial consequences to its citizens, but for global investors, it is but a blip in the Bloomberg newsfeed that moves down very quickly and will not get your investment committee perturbed.
In recent years, what did get your investment strategists or country credit risk managers worried were the European debt crisis, a rapidly increasing corporate and banking sector leverage in China, the debt ceiling debate in the US, Brexit and other confidence crises within the largest and most connected economies. A typical scenario would be that your line manager or an important client might have woken up in the morning with some headline in the FT concerning an imminent collapse of the world economy, and fired a message to you. Let’s assume for the sake of this blog that the news was “Italian banks need a capital increase from the government”. What do we think about that?
Sounds familiar? So, let’s get started working on it because you need to have a view before noon if not sooner. But where to start? How much capital is needed to bring the banking sector on stable footing? Can the government afford it? Will there be a credit crunch and fiscal tightening, which would push the economy into recession? Ultimately, is this enough to trigger a rating downgrade beyond the investment grade border and into the speculative land with all the knock on effects on investment restrictions, higher capital charges, lower lending values, changes to transfer pricing?
For most of the above questions, but especially from the point of view of a potential rating downgrade, you need to have some form of framework that allows you to analyse the complexity beyond anecdotal evidence. Let’s build a sovereign rating model to tackle this question.
Dependent variable: Sovereign debt rating
First, we need to get historical sovereign rating data for as many as possible countries, and to go back in time to have enough observations to derive a decent estimation. If you have a Bloomberg terminal in front of you, “CSDR <GO>” could be one source of data. But everyone familiar with this function would shiver since you cannot download historical data from this page or use a related BLP-function in Excel. You need to manually write the data into a spreadsheet, one country after the other. Not a fun job, or one that is fraught to exceed your midday deadline. If you have access to the research pages of the rating agencies, some of them offer downloadable rating history files (Here a link to a publicly available but outdated file from Fitch). My preferred source of sovereign rating information is from Trading Economics, which also gives you the rating history for all countries.
Gathering rating information from the big three agencies (i.e., S&P, Moody’s, Fitch) results in roughly 4,000 rating actions (rating upgrade, downgrade or confirmation and changes to the outlook). In order to make the ratings across agencies comparable (i.e. Baa1 versus BBB+), we map them into a numerical value ranging from 1 denoting AAA-rated country to 21 for a C-rated country. For countries in default or selective default, we use the numerical value 22. Alternatively, we can convert the letter rating in ordinal values that can be used with programs like Matlab, R or Python. The final step in preparing the dependent variable is to harmonize the time dimension across countries. As agencies might keep the rating of a country unchanged for some years, we need to fill the period — typically on a year-end basis — between the ratings. For instance, S&P’s rating actions over the last 5 years for Italy were:
Converting it to a common timeline and numerical values:
If you are using Matlab, we can recommend the financial time series functions “fillts” or in Python pandas are your best friends for doing this. As a result, we now have approx. 8000 rating observations and can also calculate the average rating for a specific country over time.
Explanatory variables
Of course, we have some idea about the key drivers of sovereign risk which influence its ability and willingness to honour foreign currency long-term debt obligations. A low stock of debt as a start should help, as would a balanced fiscal account, low interest expense on public debt and solid nominal economic growth. Some handy papers to support our thesis include the seminal works by Cantor and Packer “Sovereign Credit Ratings” published in 1995 by the New York Fed and the IMF’s “The uses and abuses of sovereign credit ratings”. One should not ignore the rating methodologies of the agencies to help identify explanatory variables for our little sovereign risk and rating model. As we are in a bit of rush, we identified following variables:
GDP per Capita (PPP), Real GDP Volatility (5yr), Savings to GDP, Trade Openness, Rule of Law, Control of Corruption, Government Effectiveness, Regulatory Quality, Voice & Accountability, Political Stability, CPI Average (5yr), CPI Volatility (5yr), Change in Domestic Credit to GDP, Real Interest Rate, Fiscal Balance to GDP, Tax Revenues to GDP, General Government Debt to GDP, Interest Payments (Public Debt) to Tax Revenues, Interest Payments (Public External Debt) to Exports, Current Account Balance to GDP (3yr), Export Growth (3yr), REER Deviation from Trend (5yr), Net External Debt to GDP, Net External Debt to Exports, Short-term External Debt to FX Reserves, Interest Payments (Total External Debt) to Exports, Imports to FX Reserves, External Financing Requirements
Now we need to get historical data for all indicators, countries and years that match our rating history dataset. Approximately 130 countries, 28 indicators and 40 years translate into 145,600 data points. Clearly, there is no chance to plug 150k entries manually in a spreadsheet. Bloomberg or Datastream might be the preferred source for macroeconomic data. If such data providers are out of reach, The World Bank’s open data initiative is in my view the best data source. You can easily access data using pandas remote data access functions.
Model estimation
Our task of estimating the credit rating can be described as a classification problem and we could use some type of discriminant analysis, multi-nominal logistic regression model or regression tree model. Let’s use the latter. First, let’s see which of our chosen indicators are actually useful for estimating sovereign credit ratings. Based on the feature importance for regression tree, the most important indicators are the following:
That is pretty much in line with many empirical papers, which find that institution and governance indicators and the level of wealth (i.e. GDP per capita) are among the most important drivers of ratings. In addition, indicators related to debt service capacity and overall debt ratios are also relevant factors.
Given the estimated model, we can calculate the fitted ratings for all the countries. Below you see a chart which shows the actual and estimated ratings for Italy. Overall, the model gets the rating level as well as the rating changes broadly right over time. And, we can use the model to stress test the rating given various assumptions for the future development of the explanatory indicators.
Let’s also look at the model estimated values for some other countries:
While the model works well for countries like Germany, France and even Spain. However, in the case of crisis countries such as Greece or Ireland, the model did not perform so well. It seems that other factors than in the past were driving the rating decisions during this stress period. In addition, the model focus on structural variables (e.g. quality of institutions) and level of wealth (i.e. GDP per capita) typically results in strong ratings for countries like Greece or Ireland.
Please leave a comment or send us an email if you are interested in the full results for all 130 countries in this analysis.
What are the shortcomings of such an approach?
1) Did we get the objective right?
We set out to measure sovereign risk, and we made the assumption that credit ratings of the three largest external rating agencies are the right measure for it. However, this is a heroic and misleading assumption since time and again, the rating agencies did not get it right. Effectively, we built a model explaining rating agency ratings and not necessarily sovereign risk, and we did nothing but benchmarked ourselves against them.
2) Defaults versus rating
As agency ratings are only an approximation of sovereign risk, why not directly estimate a model that replaces agency ratings with sovereign default events? The test here is that the actual number of sovereign defaults is very limited thus makes it challenging to model it. In a future post, we will write about estimating default probabilities and mapping estimated probabilities to a letter rating.
3) Holistic understanding of sovereign risk
While there are certainly common drivers of sovereign and country risks, countries are complex and requires diligent and comprehensive assessment. This requires an extensive analysis beyond looking at debt ratios, and involves an evaluation of the country’s political risks and business environment, its banking sector, external accounts and many other areas.
4) General modelling challenges
For this exercise here we took some short-cuts that we need to be fully aware. First and foremost, we fitted the model on an in-sample basis. We should have tested the stability of explanatory factors by dividing the sample into various sample periods. Secondly, we assumed that we have knowledge of the explanatory variables at the given dates. However, in most cases there are substantial reporting lags in macroeconomic data, such as The World Bank’s Governance Indicators. Thirdly, many of the explanatory indicators are correlated.
CountryRisk.io approach
In our platform, CountryRisk.io, it was very important for us to have a well-balanced model, which brings together the advantages of quantitative indicators that are automatically assessed, and qualitative indicators to broaden the analysis with country-specific characteristics in order to yield a comprehensive and holistic assessment. We follow a scoring based approach, which we find superior in understanding the many facets of the economic, social and political situation of a country and its impact on sovereign and country risk. It is our conviction that not all aspects can be captured in hard numbers (e.g. quality of fiscal management) but require a subjective assessment by the analyst. A scoring based approach allows making this process as transparent as possible. And by pooling the subjective assessments of many users for a certain qualitative indicator, we at CountryRisk.io can establish a consensus assessment. The wisdom-of-the-crowd rating provides valuable information to everyone contributing: How does my rating compare to the consensus? Is my rating higher or lower compared to the median rating? In which risk sections is my rating different from the consensus average?
CountryRisk.io provides you a comprehensive analysis framework, statistical data and access to community to exchange views on country risk analysis and specific country ratings. With CountryRisk.io you do not need to spend time on building a model and gathering (historical) data. You can focus on the analysis. Completing a rating using the CountryRisk.io model typically takes you 30 minutes so you can have an answer for your line manager just before his second coffee in the morning.
We would appreciate any comments and input about your approaches to country and sovereign risk assessments.
This post was jointly written by
Jennifer Asuncion ([email protected]) and Bernhard Obenhuber ([email protected])
About CountryRisk.io
The CountryRisk.io rating platform is open to everyone. Its rating model enables users to perform comprehensive, holistic sovereign and country risk analyses. The platform also encourages the constructive exchange of views among its users.
As members of the CountryRisk.io community, we collectively generate multiple rating views for a country based on each individual’s fundamental analysis.
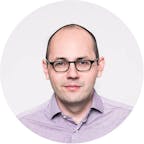